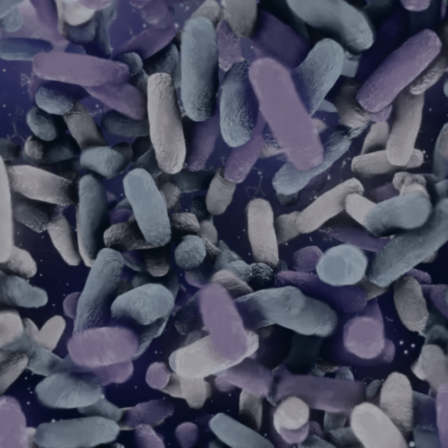
Unlike our DNA, the microbiome is dynamic and subject to frequent changes. Our research explores how the microbiome evolves over time and how these changes can indicate health and disease. Our toolkit includes tensor factorization, time-series analysis, and machine learning. We develop computational methods designed to serve the entire microbiome community and apply these methods to decipher the microbiome's role in fertility, pregnancy, and lactation.

Studying the human microbiome presents several challenges: (1) microbial communities are represented by noisy, high-dimensional metagenomic data, (2) they are dynamic and subject to frequent changes, and (3) they are complex, composed of diverse species with unique metabolic functions that require the integration of multiple data types. To address these challenges and identify robust, reproducible patterns that differentiate health-related phenotypes, we develop computational methods that perform dimensionality reduction and capture the ecological and eco-evolutionary dynamics of microbial communities.

We study how the vaginal ecosystem influence reproductive health and outcomes. By profiling the microbiomes of individuals undergoing fertility treatments, we aim to identify specific microbial patterns that correlate with successful conception and pregnancy. Our approach integrates high-dimensional metagenomic data and advanced computational methods to uncover the ecological dynamics of the microbiome, allowing us to explore how these communities interact with host physiology. Ultimately, our findings seek to inform potential therapeutic strategies that could enhance fertility and improve reproductive health.

We study how maternal microbial communities change during pregnancy and how these changes can be leveraged to predict pregnancy-related disorders and elucidate their underlying mechanisms.

We study how the infant microbiome develops in early life, in both term and preterm infants, with a special focus on the interaction between human milk composition, microbial development, and the developmental origins of disease.